Getting Started¶
Key modules and concepts¶
DIgging defines DIgger
to operate searching procedures. Users can
deploy digging by define a target_function
and SearchSpace
. The Digger
will iteratively propose new samples and update its target score yielding better
candidates.
Common digging pipeline uses a ProblemHandler
to interact with Digger
. It
will store all the searched samples and scores, ask scores of a batch of samples and
update best sample and score after each asking. The modules and workflows are shown
in the following image.
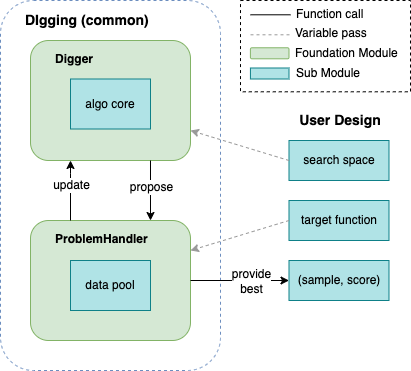
Reinforcement Learning digging pipeline needs to build a ProblemEnv
which generates
state, reward of a provided searching action, and use it to train the RL policy in Digger
.
Some other RL workers such as collector and replay buffer need to be established to execute
RL procedure. The modules and workflows are shown in the following image.
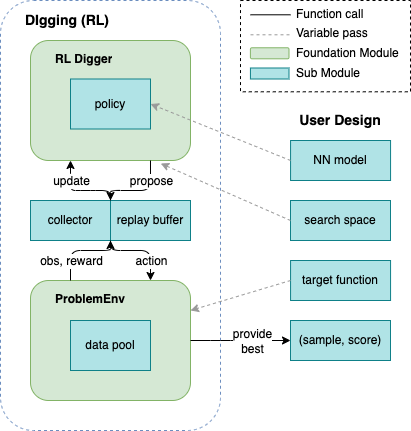
Basic Examples¶
Common digging¶
DIgging provides two kinds of searching workflow for user provided target function and space.
Interactive Procedure
It is done by calling Digger
’s propose
and update_score
method, in which you can
flexibly define the searching procedures. You can call the provide_best
method at any time
to see the currently best candidate sample and its score.
Here’s an simple example:
def target_func(x):
...
return score
space = YourSpace(shape=(...))
digger = YourDigger(config, space)
for i in range(max_iterations):
samples = digger.propose(sample_num)
scores = [target_func(x) for x in samples]
digger.update_score(samples, scores)
print(digger.provide_best())
Functional Procedure
It is done by calling the search
method of Digger
, with target function provided as input.
The digger will automatically search the best samples of the target according to the config.
Here’s an example:
def target_func(x):
...
return score
space = YourSpace(shape=(...))
digger = YourDigger(config, space)
digger.search(target_func)
print(digger.provide_best())
RL digging¶
When using a Reinforcement Learning Digger
, users need to provide an RL Policy
defined in
DI-engine form, and some other RL workers in DI-engine such as Collector
, Learner
,
ReplayBuffer
are supposed to be used in the Digger
. In the searching procedure, a target
Env
is used instead of a function. So we suggest to use the search
method to if the user
is not familiar with the RL pipeline of DI-engine. Here’s an example.
def target_func(x):
...
return score
rl_config = EasyDict(dict(...))
space = YourSearchSpace(shape=(...))
policy = YourPolicy(rl_config.policy, ...)
digger = RLDigger(rl_cfg, space, policy)
digger.search(target_func)
print(digger.provide_best())