Distributed¶
Using the Event System¶
Before we start the distributed running, let’s introduce the event system of DI-engine, which will be used by all remote calls.
The event system is an asynchronous programming paradigm that has the advantage of decoupling the code of different logics, increasing readability and, due to asynchronous execution, resource utilization. The event system also has some disadvantages, as asynchronous calls can make debugging more difficult, we will see later in the Code specification describes some ways to avoid these drawbacks.
The task object provides five methods related to the event system – emit
, on
, off
, once
, wait_for
– and we will focus on the emit
and on
methods. All other methods are derived from these two methods.
with task.start(async_mode=False, ctx=OnlineRLContext()):
task.on("greeting", lambda msg: print("Get msg: %s" % msg))
task.emit("greeting", "Hi")
# >>> Get msg: Hi
The above is a simple example of the event system, registering a callback method for the greeting
event via task.on
, triggering the event via task.emit
and sending the msg
parameter. The number of parameters is variable, as long as the emit
and on
callback function parameters correspond. Next, we split the two lines of code into separate code snippets (written in middleware form), which also work as follows.
def receiver():
# Since `on` is a permanent callback, we only need to register it once.
# If you only want to call back once, you can use `once`.
task.on("greeting", lambda msg: print("Get msg: %s" % msg))
def _receiver(ctx):
pass
return _receiver
def sender():
def _sender(ctx):
task.exit("greeting", "Hi %s times" % ctx.total_step)
return _sender
with task.start(async_mode=False, ctx=OnlineRLContext()):
task.use(receiver())
task.use(sender())
task.run(10)
This code will send and receive greeting events ten times in one process, let’s see how to use them in different processes.
Parallelize¶
The Parallel
module is a parallel module in DI-engine that will allow your main function to run in multiple processes. It integrates a message middleware internally, which, together with task’s event system, allows you to pass information between processes without sensing it.
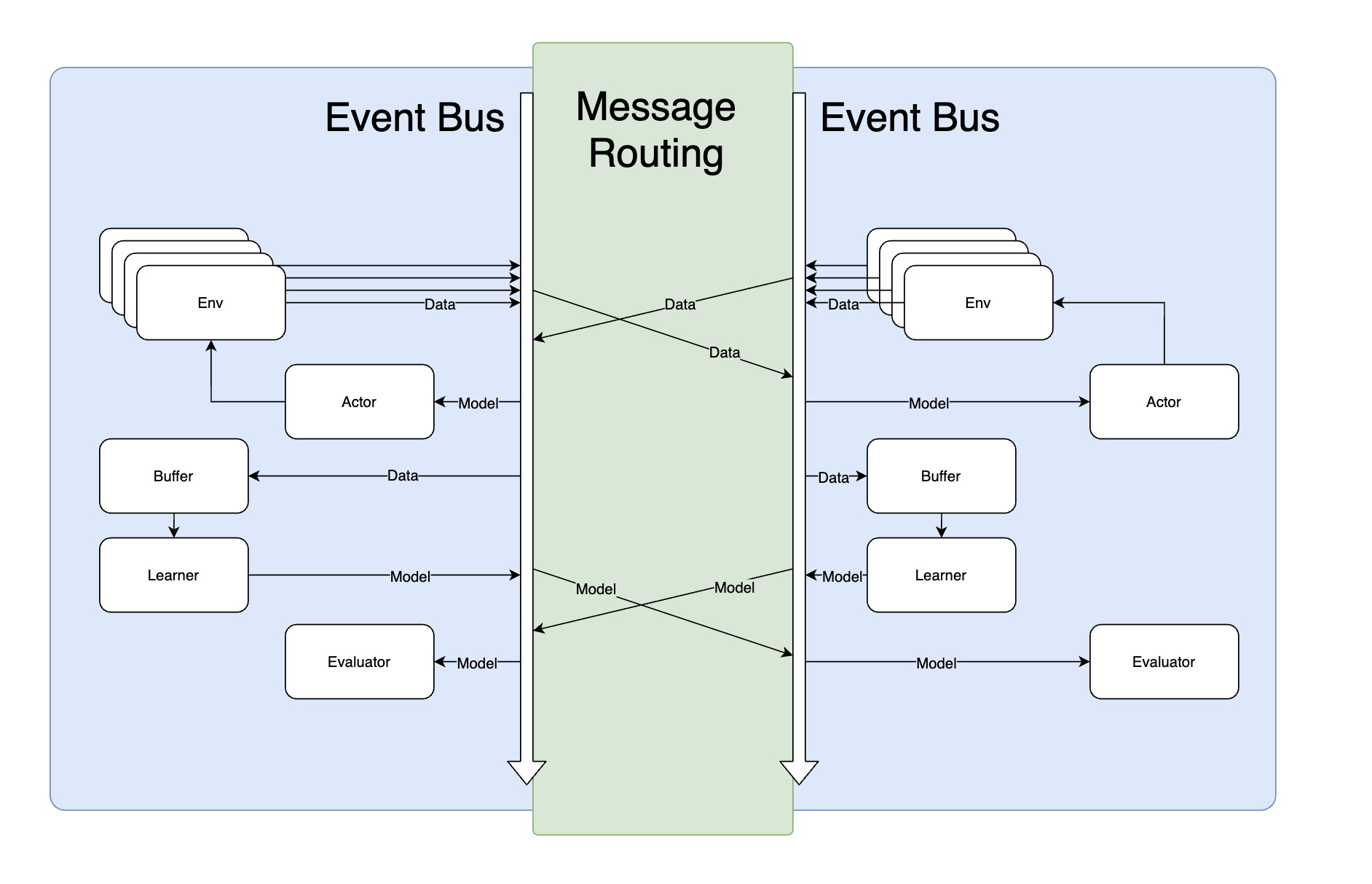
When executing code with Parallel
, the task’s internal event system will automatically route messages to connected processes. This allows you to use events and data from other processes as if you were using the event system locally.
def main():
with task.start(async_mode=False, ctx=OnlineRLContext()):
if task.router.node_id == 0: # 1
task.on("greeting", lambda msg: print("Get msg: %s" % msg))
else:
task.emit("greeting", "Hi") # 2
sleep(10) # 3
if __name__ == "__main__":
Parallel.runner(n_parallel_workers=2)(main) # 4
Note
You can access the
Parallel
instance through thetask.router
object to get the numbernode_id
of the current process, so that you can execute different function logic within different processes.You can control who sends the data via the
only_local
andonly_remote
parameters oftask.emit
, which will be broadcast to all processes by default.Since
with statement
oftask
will clear all registered events when it exits, we use sleep in the example to prevent the task from exiting prematurely.You can look at the other parameters of
Parallel
in the api documentation to choose more network modes, including mesh connections, star connections, using redis as a messaging middleware, and so on.
Deploying on Kubernetes¶
We equate distributed operation with multi-process operation, so there is no need to make any changes in the code to scale from a single to a multi-computer environment. However, we prefer to use kubernetes for DI-engine deployments to better use of task management and resource isolation techniques.
To be able to run on kubernetes with one click, we recommend using the command line tool ditask
provided by DI-engine instead of starting the application directly from a python script. ditask
supports all parameters of the Parallel
module, and you can start the application via ditask by simply completing the main function in your code.
ditask --package my_module --main my_module.main --parallel-workers 2
Using ditask, you can start multiple processes on a single machine. When we start multiple pods using kubernetes, we need to expose the ip address of all pods to each process via environment variables. To do this we provide a special kubernetes task type called DIJob
to enable the configuration of these environment variables automatically.
You can install DIJob via DI-orchestrator. Once installed, the following template can help you quickly deploy the DI-engine on kubernetes.
apiVersion: diengine.opendilab.org/v2alpha1
kind: DIJob
metadata:
name: test
spec:
minReplicas: 3
maxReplicas: 3
template:
spec:
containers:
- name: di-container
image: opendilab/ding:latest
imagePullPolicy: Always
env:
- name: PYTHONUNBUFFERED
value: "1"
resources:
requests:
cpu: 6
memory: "10Gi"
limits:
cpu: 6
memory: "10Gi"
command: ["/bin/bash", "-c",]
args:
- |
ditask --package my_module --main my_module.main --parallel-workers 2
Note
The above template will start 6 DI-engine processes (3 pods, two processes per pod)
Worker & Job & Pod & Task & Node & …¶
Since DI-engine supports standalone, k8s and slurm deployment, and k8s and slurm itself have similar concepts like node and task, here are some explanations to avoid confusion.
def main():
with task.start(async_mode=False, ctx=OnlineRLContext()):
if task.router.is_active: # In distributed mode
if task.router.node_id == 0:
... # Use learner middlewares
elif task.router.node_id == 1:
... # Use evaluator middlewares
else:
... # Use collector middlewares
task.run()
if __name__ == "__main__":
main()
The above code divides the task into 1 learner + 1 evaluator + N collector by different node_id (see dqn.py for full code). where node_id is the number of workers in the ditask, labeled 0 to N. Assuming we set the number of workers to 4, the above code will be divided into four processes in the order of learner, evaluator and 2 collectors.
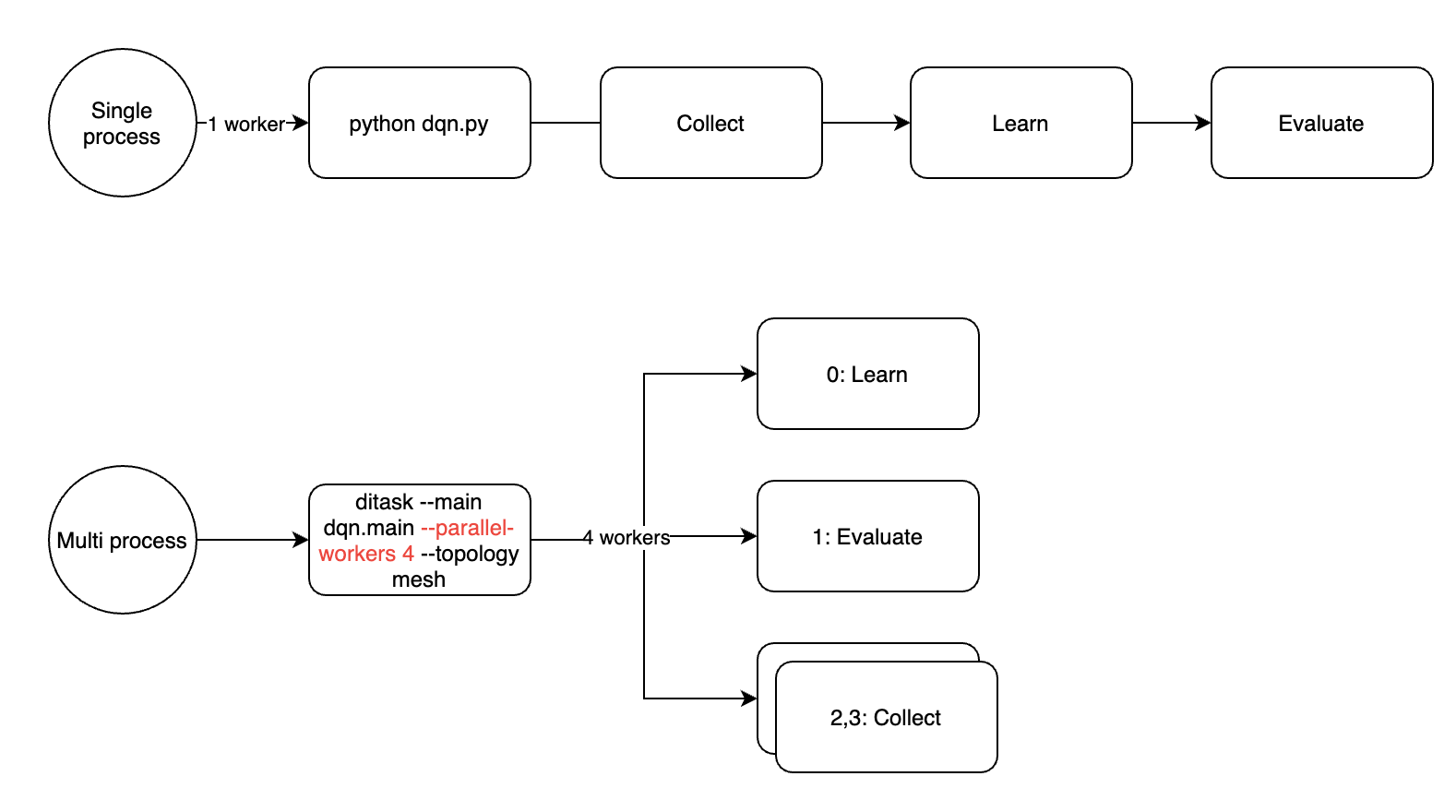
Slurm clusters bring two concepts of node and task. Node represents a cluster node, which corresponds to a physical machine, and each node can be assigned multiple tasks, which correspond to processes. So when running ditask in slurm it is recommended to enable only one worker per ditask (ditask parameter –parallel-workers 1) and the number of slurm tasks is equal to 4 (srun parameter -n 4).
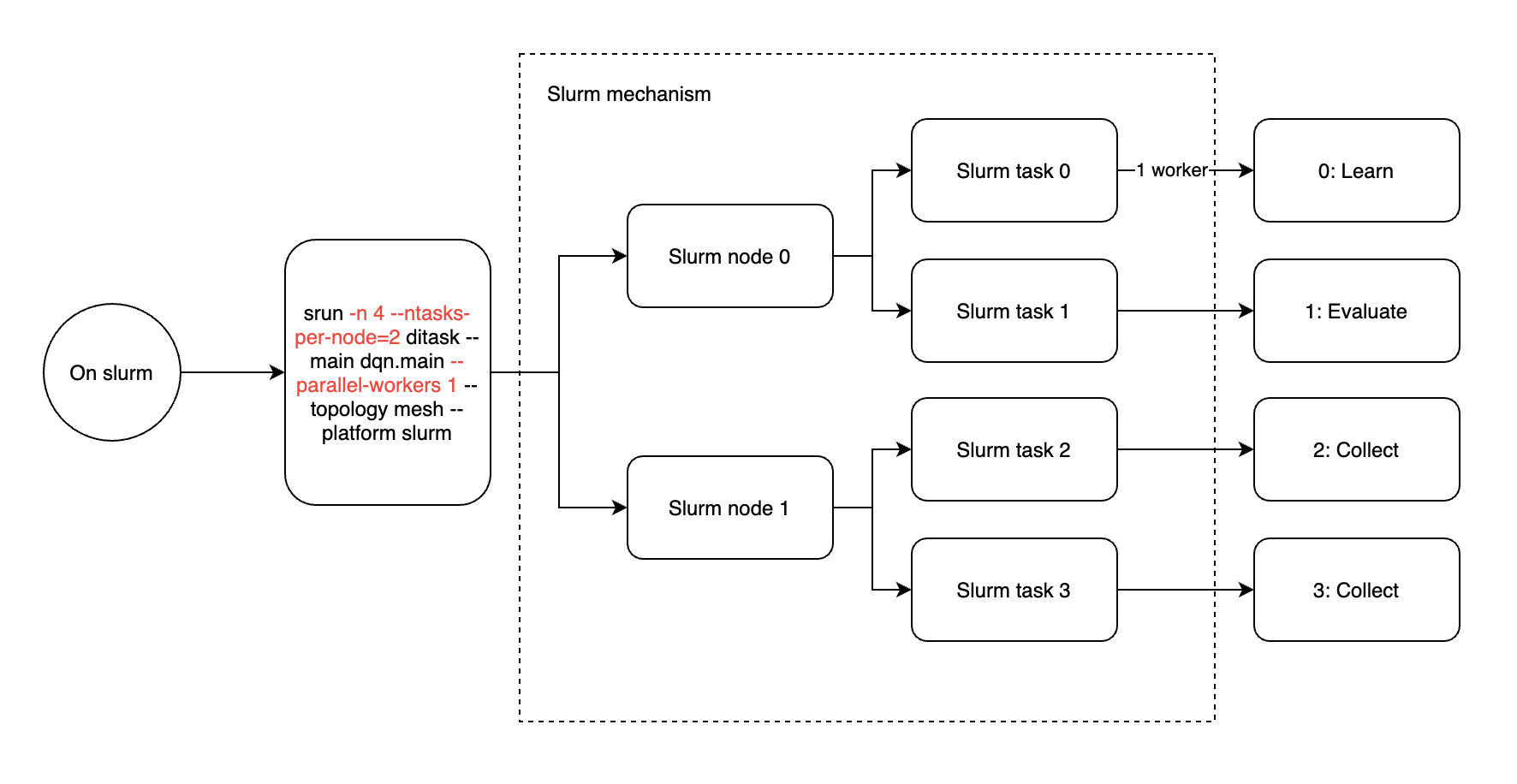
By analogy, K8s clustering brings the concept of jobs and pods, where a job can be configured with multiple pods via replica, each with a quantitative resource allocation. Here a pod is equivalent to a process within a single machine, or the concept of a task in slurm. So we recommend deploying ditask in k8s with only one worker per ditask (ditask parameter –parallel-workers 1) and 4 replicas.
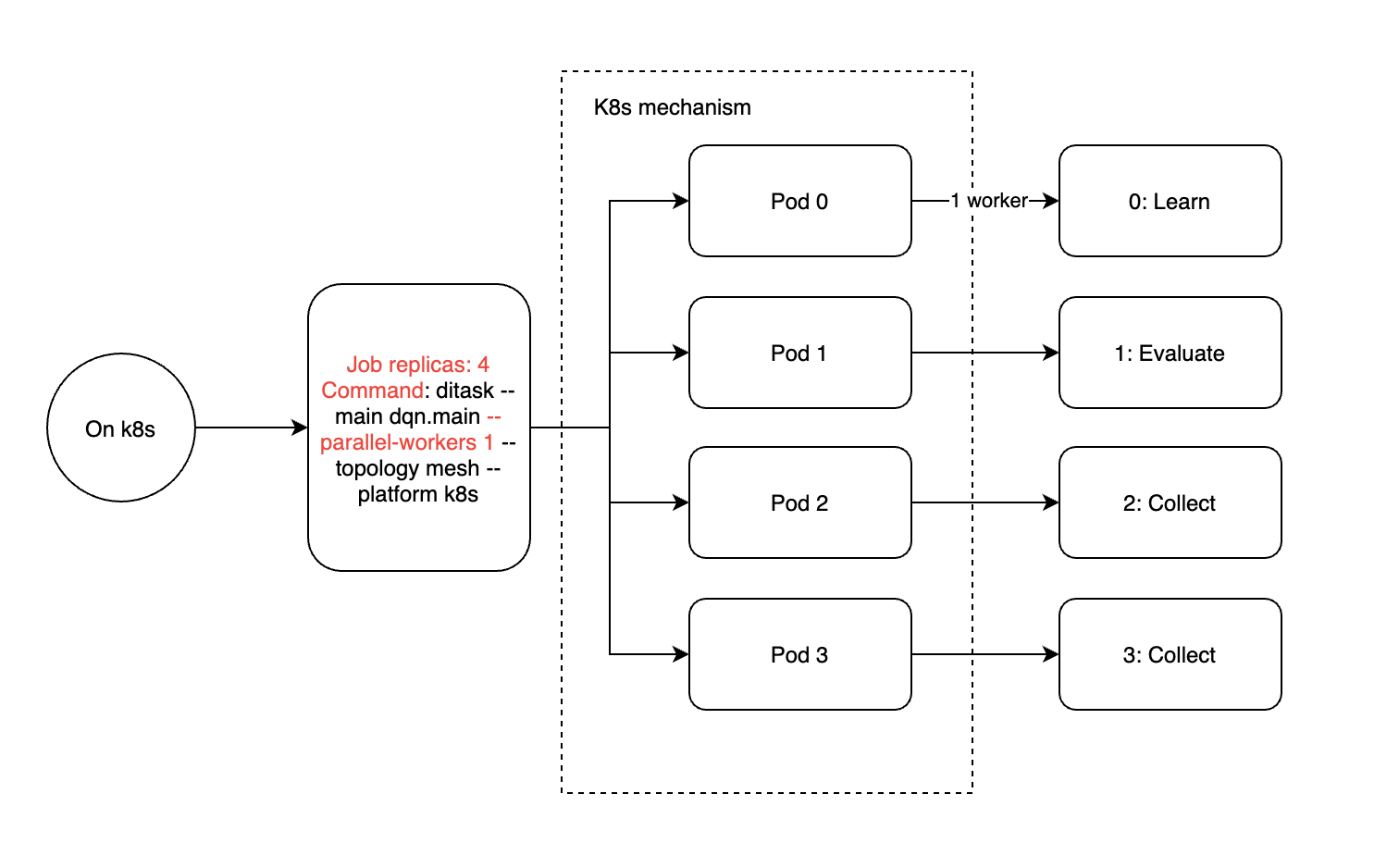
If for some special reason (e.g. you want to reduce the number of pods because you don’t have enough gpu), you can still enable multiple ditask workers in a pod of k8s or a task of slurm, and the actual executing processes will be distributed as follows. Either way, the –parallel-workers argument only affects the number of child processes in the current container, and the number of workers for the entire training task needs to be multiplied by the number of ditask master processes (number of pods or number of slurm tasks).
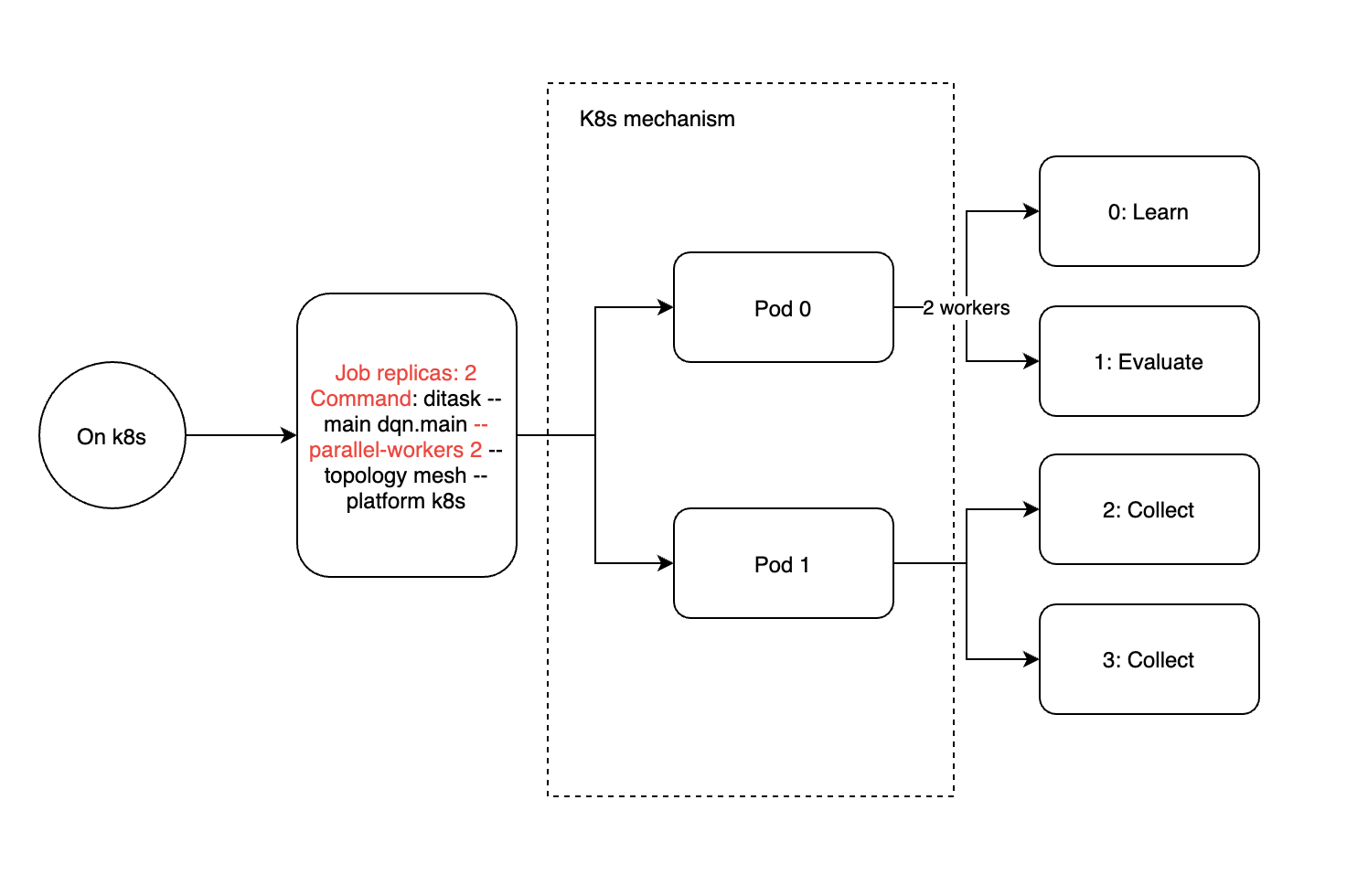