DQN¶
综述¶
传统的 Q-learning 维护一张 M*N
的Q值表(其中 M表示状态个数,N表示动作个数),通过贝尔曼方程(Bellman equation)来迭代更新 Q-value。这种算法在状态/动作空间变得很大的时候就会出现维度灾难的问题。而DQN与传统强化学习方法不同,它将 Q-learning 与深度神经网络相结合,使用深度神经网络来估计 Q 值,并通过计算时序差分(TD, Temporal-Difference) 损失,利用梯度下降算法进行更新,从而在高维空间的问题决策中(例如Atari游戏)达到了媲美甚至超过人类玩家的水平。
快速了解¶
DQN 是一个 无模型(model-free) 且 基于值函数(value-based) 的强化学习算法。
DQN 只支持 离散(discrete) 动作空间。
DQN 是一个 异策略(off-policy) 算法.
通常,DQN 使用 epsilon贪心(eps-greedy) 或 多项分布采样(multinomial sample) 来做探索(exploration)。
DQN + RNN = DRQN
DI-engine 中实现的 DQN 支持 多维度离散(multi-discrete) 动作空间,即在一个step下执行多个离散动作。
重要公示/重要图示¶
DQN 中的 TD-loss 是:
其中目标网络 \(Q_{\text {target}}\) ,带有参数 \(\theta^{-}\) ,与在线网络相同,只是它的参数会每 target_update_freq
个环境步数从在线网络复制更新一次(超参数 target_update_freq
可以在配置文件中修改。请参考 TargetNetworkWrapper 了解更多详情)。
伪代码¶
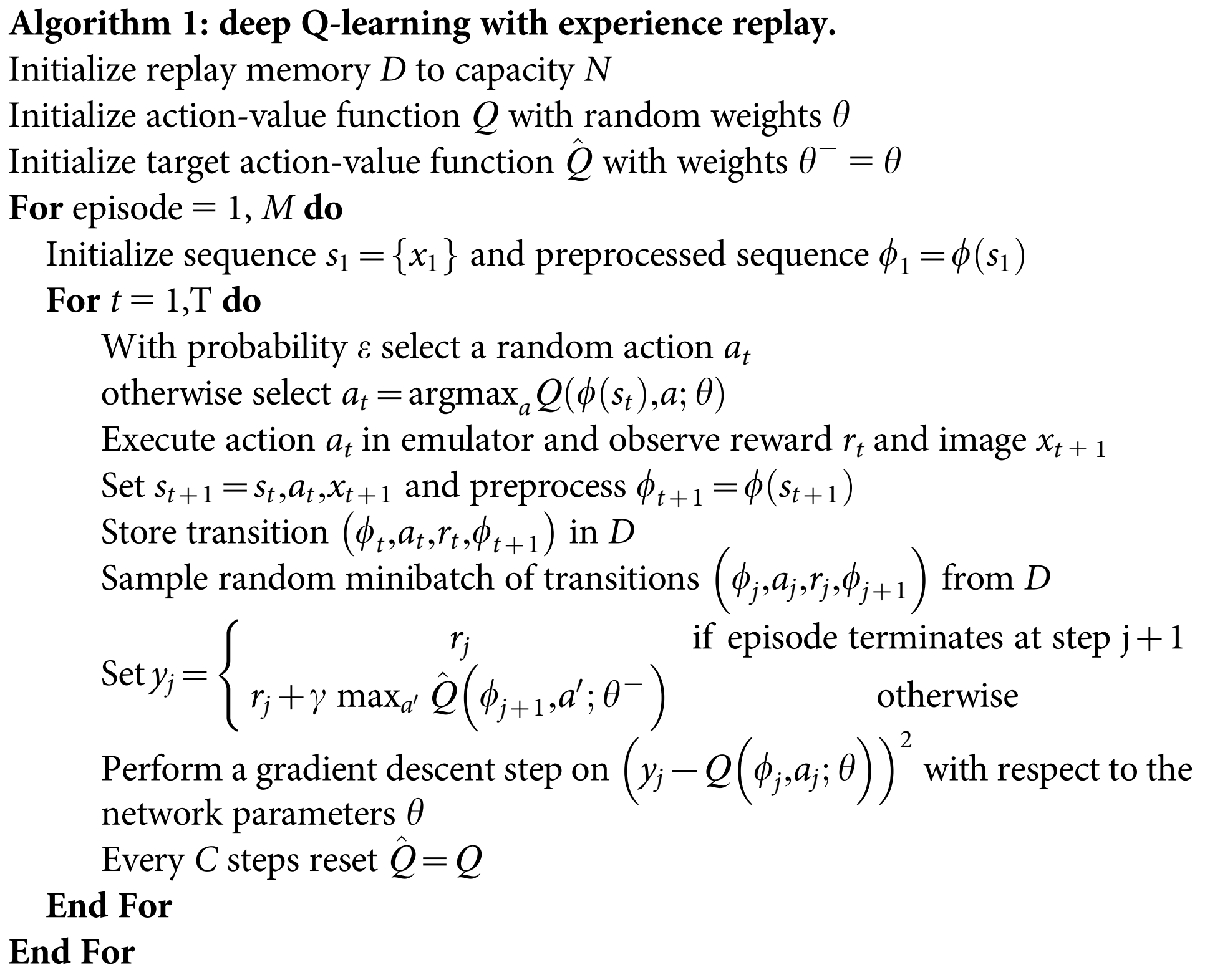
Note
与发表在 Nature 的版本相比,现代的 DQN 在算法和实现方面都得到了显著改进。譬如,在算法部分,TD-loss, PER, n-step, target network and dueling head 等技巧被广泛使用,感兴趣的读者可参考论文 Rainbow: Combining Improvements in Deep Reinforcement Learning。
扩展¶
DQN 可以和以下方法相结合:
优先级经验回放 (PER,Prioritized Experience Replay )
Prioritized Experience Replay 用一种特殊定义的“优先级”来代替经验回放池中的均匀采样。该优先级可由各种指标定义,如绝对TD误差、观察的新颖性等。通过优先采样,DQN的收敛速度和性能可以得到很大的提高。
优先级经验回放(PER)有两种实现方式,其中一种较常用方式的伪代码如下图所示:
![]()
在DI-engine中,PER可以通过修改配置文件中的
priority
和priority_IS_weight
两个字段来控制,具体的代码实现可以参考 PER code 。具体的示例讲解可以参考 PER example多步(Multi-step) TD-loss
在 Single-step TD-loss 中,Q-learning 通过贝尔曼方程更新 \(Q(s,a)\):
\[r(s,a)+\gamma \max_{a^{'}}Q(s',a')\]在 Multi-step TD-loss 中,贝尔曼方程是:
\[\sum_{t=0}^{n-1}\gamma^t r(s_t,a_t) + \gamma^n \max_{a^{'}}Q(s_n,a')\]Note
在DQN中使用 Multi-step TD-loss 有一个潜在的问题:采用 epsilon 贪心收集数据时, Q值的估计是有偏的。 因为t >= 1时,\(r(s_t,a_t)\) 是在 epsilon-greedy 策略下采样的,而不是通过正在学习的策略本身来采样。但实践中发现 Multi-step TD-loss 与 epsilon-greedy 结合使用,一般都可以明显提升智能体的最终性能。
在DI-engine中,Multi-step TD-loss 可以通过修改配置文件中的
nstep
字段来控制,详细的损失函数计算代码可以参考 nstep code 中的q_nstep_td_error
目标网络(target network/Double DQN)
Double DQN, 在 Deep Reinforcement Learning with Double Q-learning 中被提出,是 DQN 的一种常见变种。
标准 Q 学习或 DQN 中在计算目标网络时的 max 算子使用同一个的 Q 值来选择和评估动作。这使得它选择的动作的价值更有可能被高估,从而导致过度乐观的价值估计。为了防止这种情况,我们可以将选择与评估分离。更具体地说,以下两个公式展示了二者的差异:
(1)标记的Q-learning和(2)标记的Double DQN中的目标如下图所示:
![]()
区别于传统DQN,Double DQN中的目标网络不会选择当前网络中离散动作空间中的最大Q值,而是首先查找 在线网络 中Q值最大的动作(对应上面公式中的 \(argmax_a Q(S_{t+1},a;\theta_t)\)),然后根据该动作从 目标网络 计算得到Q值 (对应上面公示中的 \(Q(S_{t+1},argmax_a Q(S_{t+1},a;\theta_t);\theta'_t)\))。
综上所述,Double Q-learning 可以抑制 Q 值的高估,从而减少相关的负面影响。
DI-engine 默认实现并使用 Double DQN ,没有关闭选项。
Note
过高估计可能是由函数近似误差(近似Q值的神经网络)、环境噪声、数值不稳定等原因造成的。
Dueling head (Dueling Network Architectures for Deep Reinforcement Learning)
Dueling head 结构通过对每个动作的状态-价值和优势的分解,并由上述两个部分构建最终的Q值,从而更好地评估一些与动作选择无关的状态的价值。下图展示了具体的分解结构(图片来自论文 Dueling Network Architectures for Deep Reinforcement Learning):
在DI-engine中,Dueling head 可以通过修改配置文件中模型部分的
dueling
字段来控制,具体网络结构的实现可以参考 Dueling Head 中的DuelingHead
RNN (DRQN, R2D2)
DQN与RNN结合的方法,可以参考本系列文档中的 R2D2部分
实现¶
DQNPolicy 的默认 config 如下所示:
- class ding.policy.dqn.DQNPolicy(cfg: EasyDict, model: Module | None = None, enable_field: List[str] | None = None)[source]
- Overview:
Policy class of DQN algorithm, extended by Double DQN/Dueling DQN/PER/multi-step TD.
- Config:
ID
Symbol
Type
Default Value
Description
Other(Shape)
1
type
str
dqn
RL policy register name, refer toregistryPOLICY_REGISTRY
This arg is optional,a placeholder2
cuda
bool
False
Whether to use cuda for networkThis arg can be diff-erent from modes3
on_policy
bool
False
Whether the RL algorithm is on-policyor off-policy4
priority
bool
False
Whether use priority(PER)Priority sample,update priority5
priority_IS
_weight
bool
False
Whether use Importance SamplingWeight to correct biased update. IfTrue, priority must be True.6
discount_
factor
float
0.97, [0.95, 0.999]
Reward’s future discount factor, aka.gammaMay be 1 when sparsereward env7
nstep
int
1, [3, 5]
N-step reward discount sum for targetq_value estimation8
model.dueling
bool
True
dueling head architecture9
model.encoder
_hidden
_size_list
list (int)
[32, 64, 64, 128]
Sequence ofhidden_size
ofsubsequent conv layers and thefinal dense layer.default kernel_sizeis [8, 4, 3]default stride is[4, 2 ,1]10
model.dropout
float
None
Dropout rate for dropout layers.[0,1]If set toNone
means no dropout11
learn.update
per_collect
int
3
How many updates(iterations) to trainafter collector’s one collection.Only valid in serial trainingThis args can be varyfrom envs. Bigger valmeans more off-policy12
learn.batch_
size
int
64
The number of samples of an iteration13
learn.learning
_rate
float
0.001
Gradient step length of an iteration.14
learn.target_
update_freq
int
100
Frequence of target network update.Hard(assign) update15
learn.target_
theta
float
0.005
Frequence of target network update.Only one of [target_update_freq,target_theta] should be setSoft(assign) update16
learn.ignore_
done
bool
False
Whether ignore done for target valuecalculation.Enable it for somefake termination env17
collect.n_sample
int
[8, 128]
The number of training samples of acall of collector.It varies fromdifferent envs18
collect.n_episode
int
8
The number of training episodes of acall of collectoronly one of [n_sample,n_episode] shouldbe set19
collect.unroll
_len
int
1
unroll length of an iterationIn RNN, unroll_len>120
other.eps.type
str
exp
exploration rate decay typeSupport [‘exp’,‘linear’].21
other.eps.
start
float
0.95
start value of exploration rate[0,1]22
other.eps.
end
float
0.1
end value of exploration rate[0,1]23
other.eps.
decay
int
10000
decay length of explorationgreater than 0. setdecay=10000 meansthe exploration ratedecay from startvalue to end valueduring decay length.
其中使用的神经网络接口如下所示:
- class ding.model.template.q_learning.DQN(obs_shape: int | SequenceType, action_shape: int | SequenceType, encoder_hidden_size_list: SequenceType = [128, 128, 64], dueling: bool = True, head_hidden_size: int | None = None, head_layer_num: int = 1, activation: Module | None = ReLU(), norm_type: str | None = None, dropout: float | None = None, init_bias: float | None = None)[source]
- Overview:
The neural nework structure and computation graph of Deep Q Network (DQN) algorithm, which is the most classic value-based RL algorithm for discrete action. The DQN is composed of two parts:
encoder
andhead
. Theencoder
is used to extract the feature from various observation, and thehead
is used to compute the Q value of each action dimension.- Interfaces:
__init__
,forward
.
Note
Current
DQN
supports two types of encoder:FCEncoder
andConvEncoder
, two types of head:DiscreteHead
andDuelingHead
. You can customize your own encoder or head by inheriting this class.- forward(x: Tensor) Dict [source]
- Overview:
DQN forward computation graph, input observation tensor to predict q_value.
- Arguments:
x (
torch.Tensor
): The input observation tensor data.
- Returns:
outputs (
Dict
): The output of DQN’s forward, including q_value.
- ReturnsKeys:
logit (
torch.Tensor
): Discrete Q-value output of each possible action dimension.
- Shapes:
x (
torch.Tensor
): \((B, N)\), where B is batch size and N isobs_shape
logit (
torch.Tensor
): \((B, M)\), where B is batch size and M isaction_shape
- Examples:
>>> model = DQN(32, 6) # arguments: 'obs_shape' and 'action_shape' >>> inputs = torch.randn(4, 32) >>> outputs = model(inputs) >>> assert isinstance(outputs, dict) and outputs['logit'].shape == torch.Size([4, 6])
Note
For consistency and compatibility, we name all the outputs of the network which are related to action selections as
logit
.
实验 Benchmark¶
environment |
best mean reward |
evaluation results |
config link |
comparison |
---|---|---|---|---|
Pong
(PongNoFrameskip-v4)
|
20 |
![]() |
Tianshou(20) Sb3(20)
|
|
Qbert
(QbertNoFrameskip-v4)
|
17966 |
![]() |
Tianshou(7307) Rllib(7968) Sb3(9496)
|
|
SpaceInvaders
(SpaceInvadersNoFrameskip-v4)
|
2403 |
![]() |
Tianshou(812) Rllib(1001) Sb3(622)
|
注:
以上结果是在5个不同的随机种子(即0,1,2,3,4)运行相同的配置得到
对于DQN这样的离散动作空间算法,一般选择Atari环境集进行测试(其中包括子环境Pong等),而Atari环境,一般是通过训练10M个env_step下所得的最高平均奖励来进行评价,详细的环境信息可以查看 Atari环境的介绍文档
参考文献¶
Mnih, Volodymyr, et al. “Human-level control through deep reinforcement learning.” nature 518.7540 (2015): 529-533.
Wang, Z., Schaul, T., Hessel, M., Hasselt, H., Lanctot, M., & Freitas, N. (2016, June). Dueling network architectures for deep reinforcement learning. In International conference on machine learning (pp. 1995-2003). PMLR.
Van Hasselt, H., Guez, A., & Silver, D. (2016, March). Deep reinforcement learning with double q-learning. In Proceedings of the AAAI conference on artificial intelligence (Vol. 30, No. 1).
Schaul, T., Quan, J., Antonoglou, I., & Silver, D. (2015). Prioritized experience replay. arXiv preprint arXiv:1511.05952.