DDPG¶
概述¶
DDPG (Deep Deterministic Policy Gradient) 首次在论文 Continuous control with deep reinforcement learning 中提出, 是一种同时学习Q函数和策略函数的算法。
DDPG 是基于 DPG (deterministic policy gradient) 的 无模型(model-free) 算法,属于 演员—评委(actor-critic) 方法中的一员,可以在高维、连续的动作空间上运行。 其中算法 DPG Deterministic policy gradient algorithms 与算法 NFQCA Reinforcement learning in feedback control 相似。
核心要点¶
DDPG 仅支持 连续动作空间 (例如: MuJoCo).
DDPG 是一种 异策略(off-policy) 算法.
DDPG 是一种 无模型(model-free) 和 演员—评委(actor-critic) 的强化学习算法,它会分别优化策略网络和Q网络。
通常, DDPG 使用 奥恩斯坦-乌伦贝克过程(Ornstein-Uhlenbeck process) 或 高斯过程(Gaussian process) (在我们的实现中默认使用高斯过程)来探索环境。
关键方程或关键框图¶
DDPG 包含一个参数化的策略函数(actor) \(\mu\left(s \mid \theta^{\mu}\right)\) , 此函数通过将每一个状态确定性地映射到一个具体的动作从而明确当前策略。 此外,算法还包含一个参数化的Q函数(critic) \(Q(s, a)\) 。 正如 Q-learning 算法,此函数通过贝尔曼方程优化自身。
策略网络通过将链式法则应用于初始分布的预期收益 \(J\) 来更新自身参数。
具体而言,为了最大化预期收益 \(J\) ,算法需要计算 \(J\) 对策略函数参数 \(\theta^{\mu}\) 的梯度。 \(J\) 是 \(Q(s, a)\) 的期望,所以问题转化为计算 \(Q^{\mu}(s, \mu(s))\) 对 \(\theta^{\mu}\) 的梯度。
根据链式法则,\(\nabla_{\theta^{\mu}} Q^{\mu}(s, \mu(s)) = \nabla_{\theta^{\mu}}\mu(s)\nabla_{a}Q^\mu(s,a)|_{ a=\mu\left(s\right)}+\nabla_{\theta^{\mu}} Q^{\mu}(s, a)|_{ a=\mu\left(s\right)}\)。
Deterministic policy gradient algorithms 采取了与 Off-Policy Actor-Critic 中推导 异策略版本的随机性策略梯度定理 类似的做法,舍去了上式第二项, 从而得到了近似后的 确定性策略梯度定理 :
DDPG 使用了一个 经验回放池(replay buffer) 来保证样本分布独立一致。
为了使神经网络稳定优化,DDPG 使用 软更新(“soft” target updates) 的方式来优化目标网络,而不是像 DQN 中的 hard target updates 那样定期直接复制网络的参数。 具体而言,DDPG 分别拷贝了 actor 网络 \(\mu' \left(s \mid \theta^{\mu'}\right)\) 和 critic 网络 \(Q'(s, a|\theta^{Q'})\) 用于计算目标值。 然后通过让这些目标网络缓慢跟踪学习到的网络来更新这些目标网络的权重:
其中 \(\tau<<1\)。这意味着目标值被限制为缓慢变化,大大提高了学习的稳定性。
在连续行动空间中学习的一个主要挑战是探索。然而,对于像DDPG这样的 异策略(off-policy) 算法来说,它的一个优势是可以独立于算法中的学习过程来处理探索问题。具体来说,我们通过将噪声过程 \(\mathcal{N}\) 采样的噪声添加到 actor 策略中来构建探索策略:
伪代码¶
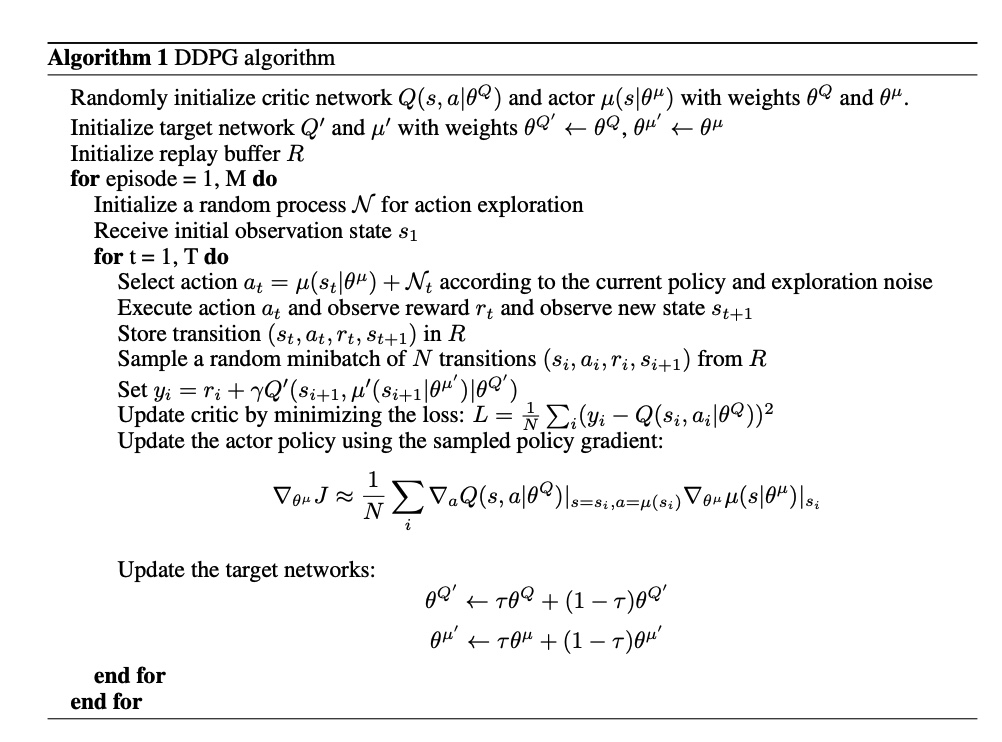
扩展¶
- DDPG 可以与以下技术相结合使用:
目标网络
Continuous control with deep reinforcement learning 提出了利用软目标更新保持网络训练稳定的方法。 因此我们通过
model_wrap
中的TargetNetworkWrapper
和配置learn.target_theta
来实现 演员—评委(actor-critic) 的软更新目标网络。遵循随机策略的经验回放池初始采集
在优化模型参数前,我们需要让经验回放池存有足够数目的遵循随机策略的 transition 数据,从而确保在算法初期模型不会对经验回放池数据过拟合。 因此我们通过配置
random-collect-size
来控制初始经验回放池中的 transition 数目。 DDPG/TD3 的random-collect-size
默认设置为25000, SAC 为10000。 我们只是简单地遵循 SpinningUp 默认设置,并使用随机策略来收集初始化数据。采集过渡过程中的高斯噪声
对于探索噪声过程,DDPG使用时间相关噪声,以提高具有惯性的物理控制问题的探索效率。 具体而言,DDPG 使用 Ornstein-Uhlenbeck 过程,其中 \(\theta = 0.15\) 且 \(\sigma = 0.2\)。Ornstein-Uhlenbeck 过程模拟了带有摩擦的布朗粒子的速度,其结果是以 0 为中心的时间相关值。 然而,由于 Ornstein-Uhlenbeck 噪声的超参数太多,我们使用高斯噪声代替了 Ornstein-Uhlenbeck 噪声。 我们通过配置
collect.noise_sigma
来控制探索程度。
实现¶
默认配置定义如下:
- class ding.policy.ddpg.DDPGPolicy(cfg: EasyDict, model: Module | None = None, enable_field: List[str] | None = None)[source]
- Overview:
Policy class of DDPG algorithm. Paper link: https://arxiv.org/abs/1509.02971.
- Config:
ID
Symbol
Type
Default Value
Description
Other(Shape)
1
type
str
ddpg
RL policy register name, referto registryPOLICY_REGISTRY
this arg is optional,a placeholder2
cuda
bool
False
Whether to use cuda for network3
random_
collect_size
int
25000
Number of randomly collectedtraining samples in replaybuffer when training starts.Default to 25000 forDDPG/TD3, 10000 forsac.4
model.twin_
critic
bool
False
Whether to use two criticnetworks or only one.Default False forDDPG, Clipped DoubleQ-learning method inTD3 paper.5
learn.learning
_rate_actor
float
1e-3
Learning rate for actornetwork(aka. policy).6
learn.learning
_rate_critic
float
1e-3
Learning rates for criticnetwork (aka. Q-network).7
learn.actor_
update_freq
int
2
When critic network updatesonce, how many times will actornetwork update.Default 1 for DDPG,2 for TD3. DelayedPolicy Updates methodin TD3 paper.8
learn.noise
bool
False
Whether to add noise on targetnetwork’s action.Default False forDDPG, True for TD3.Target Policy Smoo-thing Regularizationin TD3 paper.9
learn.-
ignore_done
bool
False
Determine whether to ignoredone flag.Use ignore_done onlyin halfcheetah env.10
learn.-
target_theta
float
0.005
Used for soft update of thetarget network.aka. Interpolationfactor in polyak aver-aging for targetnetworks.11
collect.-
noise_sigma
float
0.1
Used for add noise during co-llection, through controllingthe sigma of distributionSample noise from dis-tribution, Ornstein-Uhlenbeck process inDDPG paper, Gaussianprocess in ours.
模型¶
在这里,我们提供了 ContinuousQAC 模型作为 DDPG 的默认模型的示例。
- class ding.model.ContinuousQAC(obs_shape: int | SequenceType, action_shape: int | SequenceType | EasyDict, action_space: str, twin_critic: bool = False, actor_head_hidden_size: int = 64, actor_head_layer_num: int = 1, critic_head_hidden_size: int = 64, critic_head_layer_num: int = 1, activation: Module | None = ReLU(), norm_type: str | None = None, encoder_hidden_size_list: SequenceType | None = None, share_encoder: bool | None = False)[source]
- Overview:
The neural network and computation graph of algorithms related to Q-value Actor-Critic (QAC), such as DDPG/TD3/SAC. This model now supports continuous and hybrid action space. The ContinuousQAC is composed of four parts:
actor_encoder
,critic_encoder
,actor_head
andcritic_head
. Encoders are used to extract the feature from various observation. Heads are used to predict corresponding Q-value or action logit. In high-dimensional observation space like 2D image, we often use a shared encoder for bothactor_encoder
andcritic_encoder
. In low-dimensional observation space like 1D vector, we often use different encoders.- Interfaces:
__init__
,forward
,compute_actor
,compute_critic
- compute_actor(obs: Tensor) Dict[str, Tensor | Dict[str, Tensor]] [source]
- Overview:
QAC forward computation graph for actor part, input observation tensor to predict action or action logit.
- Arguments:
x (
torch.Tensor
): The input observation tensor data.
- Returns:
outputs (
Dict[str, Union[torch.Tensor, Dict[str, torch.Tensor]]]
): Actor output dict varying from action_space:regression
,reparameterization
,hybrid
.
- ReturnsKeys (regression):
action (
torch.Tensor
): Continuous action with same size asaction_shape
, usually in DDPG/TD3.
- ReturnsKeys (reparameterization):
logit (
Dict[str, torch.Tensor]
): The predictd reparameterization action logit, usually in SAC. It is a list containing two tensors:mu
andsigma
. The former is the mean of the gaussian distribution, the latter is the standard deviation of the gaussian distribution.
- ReturnsKeys (hybrid):
logit (
torch.Tensor
): The predicted discrete action type logit, it will be the same dimension asaction_type_shape
, i.e., all the possible discrete action types.action_args (
torch.Tensor
): Continuous action arguments with same size asaction_args_shape
.
- Shapes:
obs (
torch.Tensor
): \((B, N0)\), B is batch size and N0 corresponds toobs_shape
.action (
torch.Tensor
): \((B, N1)\), B is batch size and N1 corresponds toaction_shape
.logit.mu (
torch.Tensor
): \((B, N1)\), B is batch size and N1 corresponds toaction_shape
.logit.sigma (
torch.Tensor
): \((B, N1)\), B is batch size.logit (
torch.Tensor
): \((B, N2)\), B is batch size and N2 corresponds toaction_shape.action_type_shape
.action_args (
torch.Tensor
): \((B, N3)\), B is batch size and N3 corresponds toaction_shape.action_args_shape
.
- Examples:
>>> # Regression mode >>> model = ContinuousQAC(64, 6, 'regression') >>> obs = torch.randn(4, 64) >>> actor_outputs = model(obs,'compute_actor') >>> assert actor_outputs['action'].shape == torch.Size([4, 6]) >>> # Reparameterization Mode >>> model = ContinuousQAC(64, 6, 'reparameterization') >>> obs = torch.randn(4, 64) >>> actor_outputs = model(obs,'compute_actor') >>> assert actor_outputs['logit'][0].shape == torch.Size([4, 6]) # mu >>> actor_outputs['logit'][1].shape == torch.Size([4, 6]) # sigma
- compute_critic(inputs: Dict[str, Tensor]) Dict[str, Tensor] [source]
- Overview:
QAC forward computation graph for critic part, input observation and action tensor to predict Q-value.
- Arguments:
inputs (
Dict[str, torch.Tensor]
): The dict of input data, includingobs
andaction
tensor, also containslogit
andaction_args
tensor in hybrid action_space.
- ArgumentsKeys:
obs: (
torch.Tensor
): Observation tensor data, now supports a batch of 1-dim vector data.action (
Union[torch.Tensor, Dict]
): Continuous action with same size asaction_shape
.logit (
torch.Tensor
): Discrete action logit, only in hybrid action_space.action_args (
torch.Tensor
): Continuous action arguments, only in hybrid action_space.
- Returns:
outputs (
Dict[str, torch.Tensor]
): The output dict of QAC’s forward computation graph for critic, includingq_value
.
- ReturnKeys:
q_value (
torch.Tensor
): Q value tensor with same size as batch size.
- Shapes:
obs (
torch.Tensor
): \((B, N1)\), where B is batch size and N1 isobs_shape
.logit (
torch.Tensor
): \((B, N2)\), B is batch size and N2 corresponds toaction_shape.action_type_shape
.action_args (
torch.Tensor
): \((B, N3)\), B is batch size and N3 corresponds toaction_shape.action_args_shape
.action (
torch.Tensor
): \((B, N4)\), where B is batch size and N4 isaction_shape
.q_value (
torch.Tensor
): \((B, )\), where B is batch size.
- Examples:
>>> inputs = {'obs': torch.randn(4, 8), 'action': torch.randn(4, 1)} >>> model = ContinuousQAC(obs_shape=(8, ),action_shape=1, action_space='regression') >>> assert model(inputs, mode='compute_critic')['q_value'].shape == (4, ) # q value
- forward(inputs: Tensor | Dict[str, Tensor], mode: str) Dict[str, Tensor] [source]
- Overview:
QAC forward computation graph, input observation tensor to predict Q-value or action logit. Different
mode
will forward with different network modules to get different outputs and save computation.- Arguments:
inputs (
Union[torch.Tensor, Dict[str, torch.Tensor]]
): The input data for forward computation graph, forcompute_actor
, it is the observation tensor, forcompute_critic
, it is the dict data including obs and action tensor.mode (
str
): The forward mode, all the modes are defined in the beginning of this class.
- Returns:
output (
Dict[str, torch.Tensor]
): The output dict of QAC forward computation graph, whose key-values vary in different forward modes.
- Examples (Actor):
>>> # Regression mode >>> model = ContinuousQAC(64, 6, 'regression') >>> obs = torch.randn(4, 64) >>> actor_outputs = model(obs,'compute_actor') >>> assert actor_outputs['action'].shape == torch.Size([4, 6]) >>> # Reparameterization Mode >>> model = ContinuousQAC(64, 6, 'reparameterization') >>> obs = torch.randn(4, 64) >>> actor_outputs = model(obs,'compute_actor') >>> assert actor_outputs['logit'][0].shape == torch.Size([4, 6]) # mu >>> actor_outputs['logit'][1].shape == torch.Size([4, 6]) # sigma
- Examples (Critic):
>>> inputs = {'obs': torch.randn(4, 8), 'action': torch.randn(4, 1)} >>> model = ContinuousQAC(obs_shape=(8, ),action_shape=1, action_space='regression') >>> assert model(inputs, mode='compute_critic')['q_value'].shape == (4, ) # q value
训练 actor-critic 模型¶
首先,我们在 _init_learn
中分别初始化 actor 和 critic 优化器。
设置两个独立的优化器可以保证我们在计算 actor 损失时只更新 actor 网络参数而不更新 critic 网络,反之亦然。
# actor and critic optimizer self._optimizer_actor = Adam( self._model.actor.parameters(), lr=self._cfg.learn.learning_rate_actor, weight_decay=self._cfg.learn.weight_decay ) self._optimizer_critic = Adam( self._model.critic.parameters(), lr=self._cfg.learn.learning_rate_critic, weight_decay=self._cfg.learn.weight_decay )
- 在
_forward_learn
中,我们通过计算 critic 损失、更新 critic 网络、计算 actor 损失和更新 actor 网络来更新 actor-critic 策略。 critic loss computation
计算当前值和目标值
# current q value q_value = self._learn_model.forward(data, mode='compute_critic')['q_value'] # target q value. SARSA: first predict next action, then calculate next q value with torch.no_grad(): next_action = self._target_model.forward(next_obs, mode='compute_actor')['action'] next_data = {'obs': next_obs, 'action': next_action} target_q_value = self._target_model.forward(next_data, mode='compute_critic')['q_value']
计算损失
# DDPG: single critic network td_data = v_1step_td_data(q_value, target_q_value, reward, data['done'], data['weight']) critic_loss, td_error_per_sample = v_1step_td_error(td_data, self._gamma) loss_dict['critic_loss'] = critic_loss
critic network update
self._optimizer_critic.zero_grad() loss_dict['critic_loss'].backward() self._optimizer_critic.step()
actor loss
actor_data = self._learn_model.forward(data['obs'], mode='compute_actor') actor_data['obs'] = data['obs'] actor_loss = -self._learn_model.forward(actor_data, mode='compute_critic')['q_value'].mean() loss_dict['actor_loss'] = actor_loss
actor network update
# actor update self._optimizer_actor.zero_grad() actor_loss.backward() self._optimizer_actor.step()
目标网络¶
我们通过 _init_learn
中的目标模型初始化来实现目标网络。
我们配置 learn.target_theta
来控制平均中的插值因子。
# main and target models
self._target_model = copy.deepcopy(self._model)
self._target_model = model_wrap(
self._target_model,
wrapper_name='target',
update_type='momentum',
update_kwargs={'theta': self._cfg.learn.target_theta}
)
基准¶
environment |
best mean reward |
evaluation results |
config link |
comparison |
---|---|---|---|---|
HalfCheetah (HalfCheetah-v3) |
11334 |
![]() |
Tianshou(11719) Spinning-up(11000) |
|
Hopper (Hopper-v2) |
3516 |
![]() |
Tianshou(2197) Spinning-up(1800) |
|
Walker2d (Walker2d-v2) |
3443 |
![]() |
Tianshou(1401) Spinning-up(1950) |
P.S.:
上述结果是通过在五个不同的随机种子(0,1,2,3,4)上运行相同的配置获得的。
参考¶
Timothy P. Lillicrap, Jonathan J. Hunt, Alexander Pritzel, Nicolas Heess, Tom Erez, Yuval Tassa, David Silver, Daan Wierstra: “Continuous control with deep reinforcement learning”, 2015; [http://arxiv.org/abs/1509.02971 arXiv:1509.02971].
David Silver, Guy Lever, Nicolas Heess, Thomas Degris, Daan Wierstra, et al.. Deterministic Policy Gradient Algorithms. ICML, Jun 2014, Beijing, China. ffhal-00938992f
Hafner, R., Riedmiller, M. Reinforcement learning in feedback control. Mach Learn 84, 137–169 (2011).
Degris, T., White, M., and Sutton, R. S. (2012b). Linear off-policy actor-critic. In 29th International Conference on Machine Learning.