MetaDrive Macro Environment¶
MetaDrive Macro Environment is a control option based RL environment on MetaDrive. It is similar with highway-env, in which the ego vehicle aims to keep a high velocity on a multi-lane highway with some slower vehicles. The env use a top-down view as input and outputs a discrete macro action. The macro action takes effect in a following time period in simulator, and the vehicle is drived by a IDM policy that can achieve the target macro action.
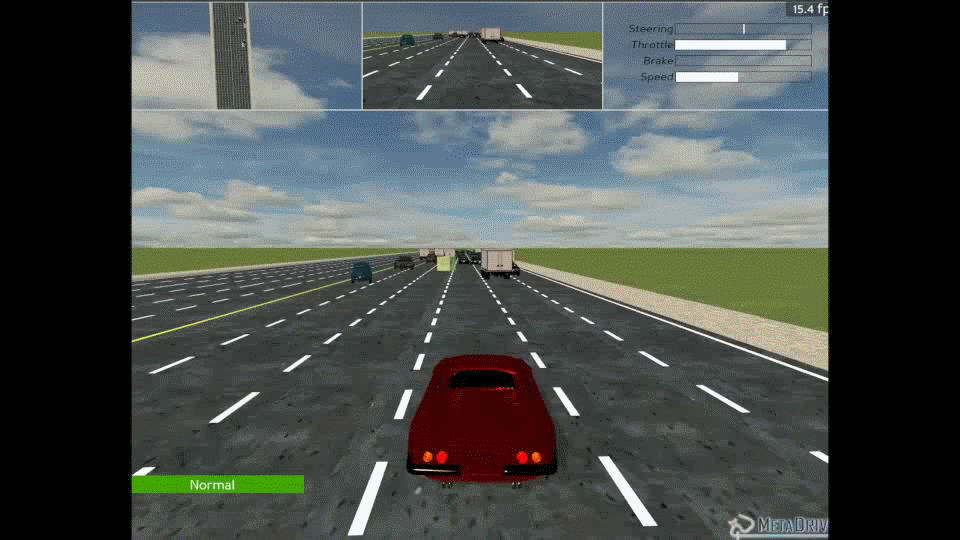
Environments¶
The environment is established following the standard gym.Env
form. Detail information
is listed below.
The state of macro env is Top-down Sematic Maps containing 5 channels:
Road and navigation
Ego now and previous position
Neighbor at step
t
Neighbor at
t-1
Neighbor at
t-2
All map elements are in the same relative coordinate system.
Reward:
driving reward: driving distance along the lane.
speed reward: the faster, the larger
termination reward: containing success and failure case
Action:
Speed up
Slow down
Change lane left
Change lane right
Hold speed
Each action costs 30 system timestep, and is converted to control signals through a PID controller.
Training & Test¶
All the entry can be found in demo/metadrive
DI-drive provide two RL policy training entries: DQN and Discrete PPO. Run the following scripts to start training DQN demos.
cd demo/metadrive
python macro_env_dqn_train.py
You may need to modify the collector and evaluator nums according to your resources.
metadrive_macro_config = dict(
env=dict(
collector_env_num=14,
...
),
...
)
After training, you can visualize the performance by running the following script:
python macro_env_dqn_eval.py
You may need to change the checkpoint path in config. Here we provide a pre-trained DQN weights as example.